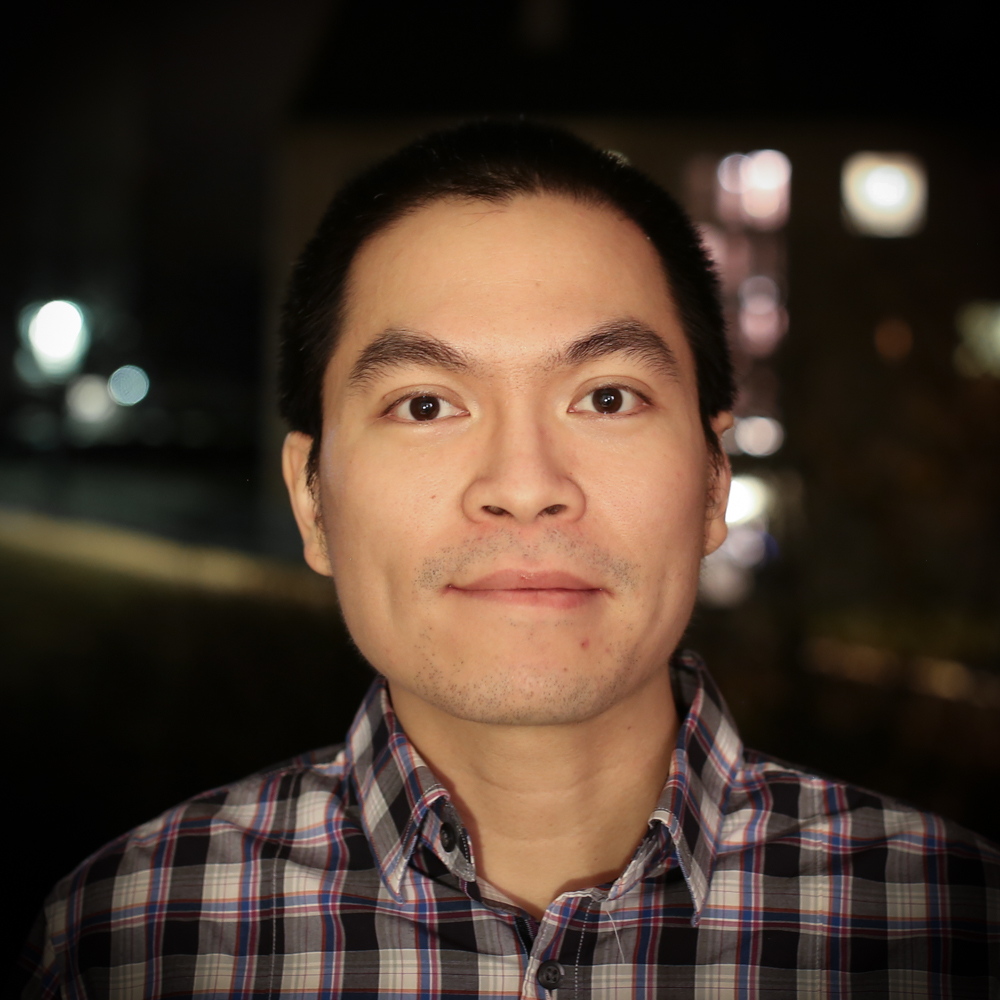
Jerry Tjiputra
Leader: Jerry Tjiputra
Objectives
To determine the evolution of “internal climate variability” and “model uncertainty” when projected into various future scenario and elucidate how the contributing key processes response to long-term anthropogenic forcings.
Background
A prominent challenge in climate science is to produce the best assessment of how the real-world climate would change under given future scenarios (Collins, 2017). In fact, the largest uncertainties in future climate projection over time scale larger than few decades is related to the selected scenario, e.g., anthropogenic CO2 scenarios (Hawkins and Sutton, 2009).
Characterizing this uncertainty, which is important for regional adaptation planning (Cox and Stephenson, 2007), starts with better understanding of the underlying processes (WP2) and our ability to constraint it in models (WP3). The next step is to determine how these ‘internal process’ and ‘constraint’ might evolve under the transient future anthropogenic influences. Findings from WP4 will also feed back to the observational community in evaluating whether the necessary monitoring networks are in place.
Description of work
Task 4.1 Analysis of internal variability evolution in CMIP6 models under forced climate change. Determine how dominant internal climate mode variability such as ENSO, NAO (North Atlantic Oscillation), SAM (Southern Annular Mode), etc. may evolve in the 21st century. Identify if there are any mechanistic consistencies in the simulated changes in the different CMIP6 models.
Task 4.2 Determine capacity for detecting thresholds and hot spots. Based on the identified mechanisms and regions of significant future change, evaluate the detectability important changes given the current observational network. Identify “heritage data sets” that are not in place today but would be a necessary reference for comparison with new data sets decades from now.
Task 4.3 Produce optimized projection of key ECVs under IPCC-defined future scenarios. Knowledge from WP2 and WP3 will provide guidelines for identifying models best suitable for predicting future climate change regionally and globally. Model weighting will be applied to the pre-selected model ensemble to arrive at “best possible” projections of key climate metrics, such as temperature, precipitation, sea-ice, carbon cycle, etc. Comparison with of different model versions (e.g., in CMIP5 vs. CMIP6) will also be conducted to provide insights of any progress in climate models.