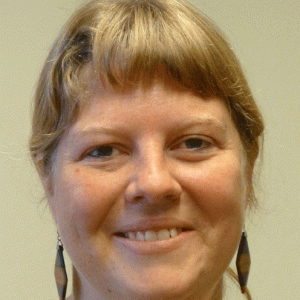
Nadine Goris
Leader: Nadine Goris
Co-leader: Jörg Schwinger
Objective
To identify best possible emergent constraints based on broad range of observational fields and to develop a systematic weighting scheme for CMIP6 models using these constraints.
Background
“Emergent constraints” is done through identifying quasi-linear relationships across an ensemble of models, typically between (i) certain aspect of long-term or future metrics in ESM and (ii) an observable trend or variation in the contemporary climate. Both terms (i) and (ii) refer to key future and present climate indices, typically with large inter-model spread, but term (ii) is constrainable with the available observations. In order to optimize the search for emergent constraint, we will therefore search along two streamlines that have been proven successful before. The first approach focuses on identifying regions whose contemporary state is important for an accurate future projection as done in the pilot-study for the COLUMBIA-project (see Proof-of-concept-Box).
The second approach focuses on identifying short-term process dynamics that are important for the long-term evolution. Several studies have shown that the simulated strength of mechanisms that are responsible for the long-term evolution of a certain variable, tend to act also on shorter time-scales (i.e. within the annual cycle), allowing to constrain the strength of each process via simple measures of the short-term dynamics (i.e. the amplitude of the annual cycle; Kessler and Tjiputra, 2016; Goris et al., in prep.).
The CMIP model ensembles are “ensemble of opportunity”, i.e., they are composed of models with varying skills and complex interdependencies (i.e., they are not equally credible, and well distributed around the ‘true real-world’). Therefore, considering each model projection to be equally valid, and averaging them to arrive at a ‘best estimates’ leads to biases and less credible climate prediction.
Both spatial and temporal emergent constraints identified in this WP will be utilised for different weighting strategies that will range from a simple 0/1 approach (i.e. leaving unsuitable models out of the future projections) to a differentiated weighting approach where each model gets assigned a value between 0 and 1 based on their contemporary skills.
Description of work
Task 3.1. Regional emergent constraints. For each of the considered variables (oceanic heat and carbon uptake and transport and ENSO variability), we will identify regions whose contemporary state is important for an accurate future projection. We will follow this approach not only variable-wise, but we will furthermore study, for which regions and depths the ocean carbon and heat storage as well as heat and carbon transport is important for the global oceanic heat and carbon uptake. For ENSO, we will study the regional influence of sea surface temperature and surface winds separately.
Task 3.2. Temporal emergent constraints. The temporal approach focuses on identifying short-term process dynamics that are important for the long-term evolution. Here, we will focus in the annual cycle for heat and carbon uptake by the oceans and on inter-annual and decadal variability for ENSO. For the heat- and carbon-related processes, we will focus on assessing the ventilation processes in the ocean using observed inventories of passive tracers, such as CFCs (Matsumoto et al., 2004), in addition to other biogeochemical tracers (Lauvset et al., 2016). For the ENSO, updated atmospheric reanalysis datasets will be used (e.g., ECMWF, Dee et al., 2011). We will evaluate to what degree certain multi-model constraints are robust across different time scales or climate states.
Task 3.3 Model weighting. We will use the regional and temporal emergent constraint as identified in Task 3.1 and 3.2 to objectively assign to each model a skill-score between 0 and 1. We will use the identified skill scores for a model weighting in order to achieve an optimized future projection. As a test, model weighting will be applied over the early historical period (e.g., up to year 1990), and optimized projections over the next decades will be determined and compared to more recent observations to validate, evaluate, and calibrate the performance improvements of our approach. Based on this, the calibrated approach will be applied on future scenarios.